A/B testing is the secret weapon behind smarter decisions in marketing, product design, and user experience. Instead of guessing what works, businesses use controlled experiments to see which version of a webpage, email, or ad drives better results. When done right, it removes the guesswork and replaces it with clear, data-backed insights.
This article breaks down A/B testing into simple steps, from choosing what to test to analyzing results. You’ll learn how to avoid common mistakes, pick the right tools, and make changes that actually move the needle.
If you’re ready to make decisions with confidence, let’s get started.
15-Step Guide to Running an A/B Test (Before, During, and After)
A well-structured A/B test follows a clear process to ensure reliable, data-driven results. Here are 15 steps to guide you through every phase—before, during, and after the test—so you can make confident, informed decisions.
Before the Test: Setting Up for Success
1. Define Your Objective
Every A/B test should start with a clear goal. Without a defined objective, you’re shooting in the dark, and even if one version performs better, you won’t know why. Are you trying to increase signups, get more people to click your CTA, or increase product purchases?
The more specific your objective, the easier it is to measure results and draw meaningful conclusions. A vague goal like “increase engagement” isn’t useful, define exactly what engagement means (e.g., time on page, button clicks, conversions).
A well-defined objective helps keep your test focused and prevents unnecessary changes. Instead of testing everything at once, prioritize elements that directly impact the desired outcome.
For example, if you want higher email signups, test your form placement or CTA wording, not the background color of the page. A test without a goal is just a random experiment, it won’t help you make better decisions.
2. Identify What to Test
A good A/B test focuses on one variable at a time. Testing too many elements at once leads to unclear results because you won’t know what actually caused the change.
Start by identifying high-impact elements, these are the things that directly influence user behavior. Examples include headlines, CTA buttons, images, layouts, pricing structures, and page speed.
Choosing what to test depends on your goal. If you want to improve click-through rates, test your headlines or CTA buttons. If you’re optimizing for purchases, experiment with pricing display, product descriptions, or checkout process.
Always ask yourself: Does this element play a role in the user’s decision-making process? If not, it’s not worth testing.
3. Develop a Hypothesis
A hypothesis is your educated guess about what will happen and why. It sets expectations and gives the test a clear direction. Instead of randomly changing elements, base your hypothesis on data, user behavior, or design psychology. A strong hypothesis follows this format: “If we change [X], then [Y] will happen because [reason].”
Without a hypothesis, it’s impossible to determine if a test was successful or just a coincidence. For example, instead of testing a random CTA color, develop a hypothesis based on contrast and visibility: “If we make the CTA button red instead of blue, we expect higher clicks because red triggers urgency and stands out more.” This approach ensures every test is purpose-driven, not guesswork.
4. Choose Your A and B Variants
A/B testing requires two versions:
- Version A (Control): The existing version, which serves as the baseline.
- Version B (Variation): The new version, which includes one specific change.
Your variation should be significant enough to measure a difference, but not so extreme that multiple factors are altered at once.
If you change the CTA, page layout, and pricing structure at the same time, you won’t know which change caused the improvement (or decline). Keep it simple, test one major difference per experiment.
5. Select the Right Audience
The effectiveness of your A/B test depends on who sees it. Your audience should be divided randomly and evenly to prevent bias. If you’re testing a change on all visitors, make sure both new and returning users are equally represented.
If the test is specific (e.g., targeting mobile users), segment accordingly to ensure the right group is analyzed.
Audience selection also depends on your business model. An e-commerce store might segment users by shopping behavior, while a SaaS business might test different pricing for new users only. The more relevant your audience, the more accurate your results.
6. Decide on the Sample Size
Testing with too few users leads to unreliable data. A/B tests need a large enough sample size to be statistically significant, meaning the results aren’t just random chance. If you test on only 100 users and see a 5% increase, that’s not meaningful, but if 50,000 users show a consistent 5% increase, that’s reliable.
Use an A/B testing calculator to determine the right sample size for your goal. More traffic allows faster testing, but if your traffic is low, you’ll need to run the test for a longer period to collect meaningful data.
7. Choose the Right A/B Testing Tool
Manually running A/B tests is a nightmare, which is why tools exist to automate the process. Popular platforms like Google Optimize, Optimizely, and VWO allow businesses to split traffic, track interactions, and measure results easily.
The right tool depends on your budget, website traffic, and integration needs. Free tools like Google Optimize are great for small businesses, while advanced tools like Optimizely offer deeper insights for larger teams. Choose one that fits your growth stage.
During the Test: Running a Controlled Experiment
8. Set Up the Test Properly
Before launching, double-check that both versions function correctly. A broken test means wasted time and misleading results.
Ensure that the new variant loads properly, tracks data accurately, and doesn’t interfere with other website elements. This step is crucial because even a simple tracking issue can invalidate the test.
Testing environments matter too. If you’re running an A/B test on a checkout page, make sure real transactions are processed smoothly. If testing an email campaign, verify that all links work and images display correctly.
Minor mistakes can drastically affect the results and lead to faulty conclusions.
9. Launch the Test and Let It Run
Once your test is live, resist the urge to interfere. Stopping an experiment too early can lead to misleading data, as results fluctuate significantly in the beginning. Many marketers panic when they see one version winning early, but results often stabilize over time.
The best practice is to let the test run for a full business cycle (at least a week, ideally longer). This helps capture a variety of user behaviors, weekday shoppers, weekend browsers, mobile vs. desktop users, etc.
Making premature decisions can ruin the accuracy of the test.
10. Monitor Key Metrics
It’s easy to get caught up in early results, but reacting too soon can lead to bad decisions. A/B tests fluctuate in the beginning, and patterns take time to stabilize.
Instead of checking every minor change, focus on tracking performance until the test reaches statistical significance. Premature adjustments can skew data and invalidate results.
Key performance indicators (KPIs) should align with your original goal. If the test is for email subject lines, open rates matter more than click-through rates. If it’s for a landing page, conversion rate is the priority, not bounce rate.
Sticking to one primary metric keeps your analysis meaningful and prevents distractions.
11. Check for Statistical Significance
Just because one version is slightly ahead doesn’t mean it’s truly the winner. Statistical significance ensures that results aren’t just random luck. If a test isn’t statistically valid, rolling out the “winner” could be a mistake that actually hurts performance.
Most A/B testing tools calculate significance automatically, so check if the confidence level is at least 95% before making a decision. If not, let the test run longer or increase the sample size. A rushed decision is worse than waiting for accurate data.
After the Test: Analyzing and Implementing Insights
12. Analyze the Results
Once the test concludes, dig into the real impact of the change. Did the variation actually improve conversions, or was the difference too small to matter? Look beyond just which version won, analyze trends, user behaviors, and consider if external factors influenced the results.
Results should align with the original hypothesis. If not, consider why. Did the test run long enough? Was the audience segmented properly? Sometimes, no result is still a result, even if the control wins, you’ve learned what doesn’t work, which is just as valuable.
13. Implement the Winning Variation
If the variation significantly outperforms the control, roll it out as the new standard. However, implementation isn’t just flipping a switch, it’s making sure the change aligns with your overall strategy. Before rolling out a new version, confirm it integrates well with the rest of the user experience.
If results are inconclusive, don’t scrap the test. Instead, refine the approach and test again. Maybe the wrong metric was used, or a different audience segment would react differently. The key is to apply learnings, not just chase instant wins.
14. Document Your Findings
Every test should be recorded for future reference. Keeping a database of A/B test results prevents teams from repeating failed experiments and helps guide future testing. This is especially useful for larger teams, where multiple people handle different aspects of optimization.
Your documentation should include:
- The test objective
- The hypothesis
- The control and variation details
- The results and statistical significance
- Key takeaways and next steps
15. Keep Testing and Iterating
A/B testing isn’t a one-and-done process. Even after rolling out a winning variation, user behaviors change, market trends evolve, and new opportunities emerge. Companies that continuously test and refine stay ahead of the competition, while those who assume they’ve “figured it out” get left behind.
The best A/B testing strategies prioritize ongoing improvement. Instead of running one-off tests, create a culture of experimentation where every major decision is backed by data. Over time, these insights compound, leading to massive business growth.
Why Is A/B Testing Important?
With countless businesses vying for attention, making the wrong design, copy, or strategy decisions can mean lost opportunities. This is where A/B testing shines, it ensures you’re not leaving success to chance. Here are a few key benefits of A/B Testing
Increased Conversion Rates
Every element of your website, email, or ad influences how people respond. A/B testing helps you identify the winning combination that drives more clicks, purchases, or sign-ups. For example, simply changing the color of a CTA button has been shown to increase conversion rates significantly.
Data-Driven Decision Making
Forget relying on your gut instinct or personal preferences. A/B testing provides hard evidence of what works and what doesn’t. This minimizes guesswork and ensures that your marketing strategies are optimized for performance.
Improved User Experience (UX)
By testing different layouts, messaging, or features, you can identify what your audience prefers. This results in a smoother, more enjoyable experience for your users, and happier users are more likely to stick around.
Better ROI on Marketing Efforts
A/B testing ensures that your campaigns aren’t wasting resources on ineffective strategies. Instead, every dollar you spend is backed by insights into what truly resonates with your audience.
A/B Testing Tools
To successfully run an A/B test, you need the right tools and resources to simplify the process, collect reliable data, and analyze your results effectively. With so many options available, it’s important to choose the ones that suit your needs and expertise level.
Here’s a breakdown of the top A/B testing tools and additional resources to help you get started.
- Google Optimize
- Best for: Beginners and small businesses.
- Features:
- Easy integration with Google Analytics.
- Free version with essential testing capabilities.
- Allows you to run A/B, multivariate, and redirect tests.
- Optimizely
- Best for: Mid-sized to enterprise-level businesses.
- Features:
- Advanced experimentation platform.
- Personalization capabilities and multivariate testing.
- Real-time results and audience targeting.
- VWO (Visual Website Optimizer)
- Best for: All business sizes looking for user-friendly tools.
- Features:
- Drag-and-drop editor for creating test variations.
- Heatmaps, session recordings, and behavioral insights.
- A/B, split URL, and multivariate testing.
- Crazy Egg
- Best for: Businesses focused on user experience and behavior.
- Features:
- Heatmaps, scrollmaps, and session recordings.
- Easy-to-use A/B testing features.
- Insights on where users click and drop off.
- Unbounce
- Best for: Marketers focused on landing page optimization.
- Features:
- Drag-and-drop landing page builder with A/B testing tools.
- Pre-built templates and AI recommendations.
- Lead generation features (like forms and pop-ups).
- Adobe Target
- Best for: Enterprise-level businesses with complex needs.
- Features:
- AI-driven personalization and testing.
- Advanced audience segmentation.
- Multivariate and A/B testing at scale.
- Kameleoon
- Best for: Companies looking to combine testing with AI-driven personalization.
- Features:
- Real-time data collection and segmentation.
- Predictive targeting with AI.
- A/B, multivariate, and predictive testing.
A/B Testing: Final Thoughts
A/B testing isn’t just a one-time experiment, it’s a mindset that leads to smarter decisions and better results. By following a structured approach, you can eliminate guesswork, optimize key elements, and continuously improve performance.
Every test, successful or not, provides valuable insights that refine your strategy. Keep testing, keep learning, and let data guide your next big win.
Frequently Asked Questions
How do you determine a winner in an A/B test?
A winner is determined by statistical significance, meaning the results are not due to chance. Most A/B testing tools calculate this automatically, usually aiming for at least a 95% confidence level. Without statistical significance, the results may not be reliable.
Why is it important to test only one change at a time in an A/B test?
Testing one change at a time ensures you know exactly what influenced the outcome. If multiple elements are changed, it becomes difficult to pinpoint which adjustment improved or hurt performance. Keeping tests simple leads to clearer, more actionable insights.
What should you do if an A/B test fails to show improvement?
If an A/B test does not improve performance, analyze if the tested element had a meaningful impact. External factors or poor test execution can sometimes affect results. Instead of stopping, refine the hypothesis and run another test with a different variation.
Related:
- The Importance of a User Experience Focus For Websites
- Google is Introducing a New User Experience Signal
- Web Design Trends That You Should Follow This Year
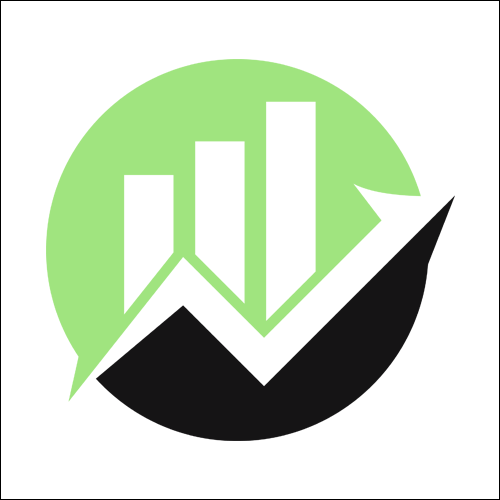
We empower people to succeed through information and essential services. Do you need help with something? Contact Us.
Want a heads-up once a week whenever a new article drops?